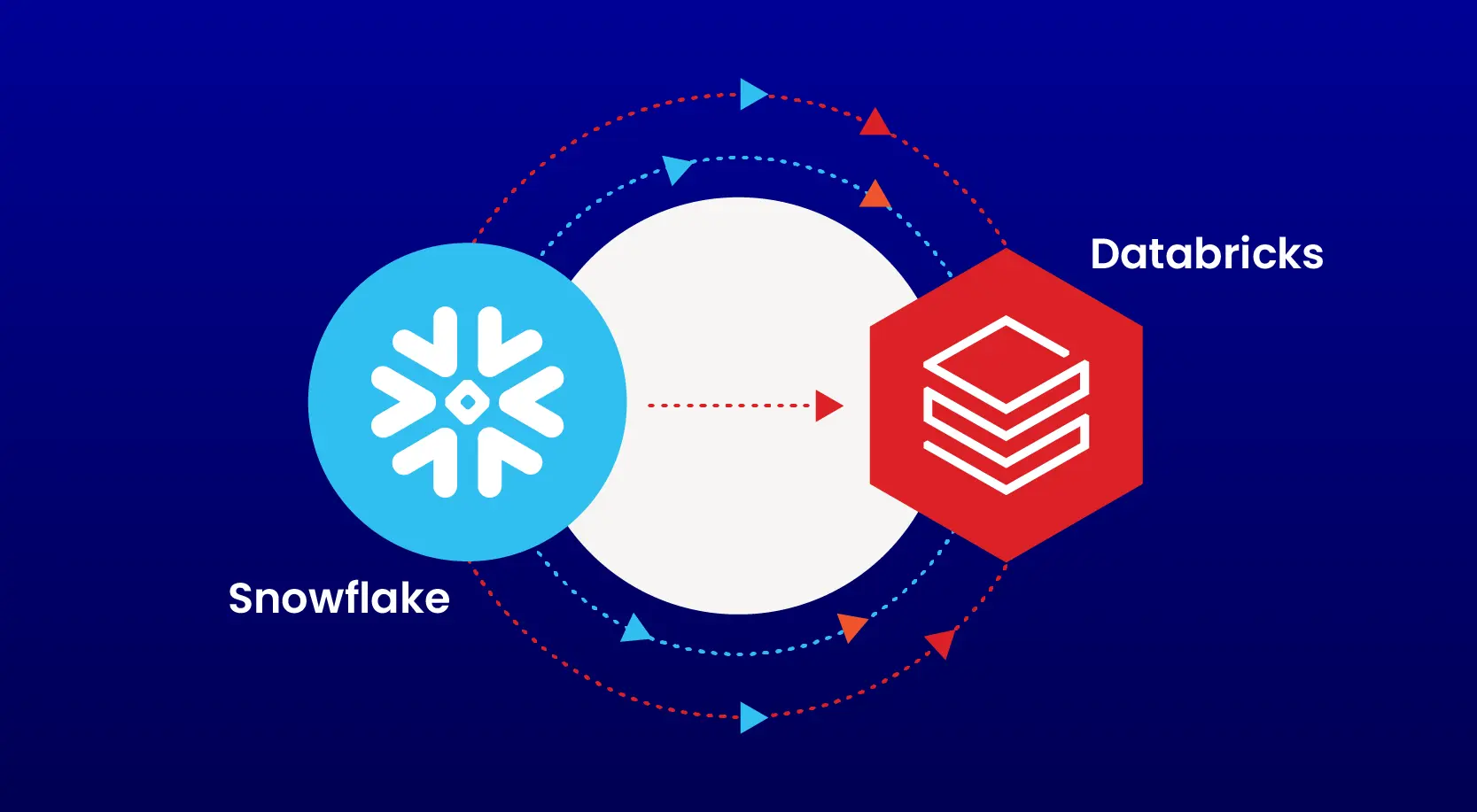
Every enterprise is a data enterprise today, and in today’s data-driven world, organizations constantly seek ways to optimize their data platforms to enable faster insights, reduce costs, and leverage cutting-edge technologies.
Snowflake is purpose-built for data warehousing, so many enterprises have adopted it as their choice of cloud data platform. However, some are now considering a shift to Databricks. At the same time, this migration may initially seem counterintuitive, but significant benefits position Databricks as a top choice for organizations aiming to leverage their data assets fully.
In this blog, we’ll explore the reasons behind migrating from Snowflake to Databricks, major advantages of the Databricks Lakehouse platform, and discuss how a Systems Integration (SI) partner like us, WinWire, can support the transition effectively.
There are two compelling factors for choosing Databricks from reputed cloud data warehouses:
- Reduce TCO: A cloud data warehouse like Snowflake is not typically optimized for data engineering workloads. The support for semi-structured and unstructured data is limited, complex, and often involves third-party tools and multiple stages. Their support for streaming is limited, too. Also, the enterprises are locked in with expensive proprietary formats.
- Accelerate ROI with innovation: A cloud data warehouse typically isn’t optimized for data science. It often involves data duplication to data lake before third-party model serving takes over. Meanwhile, these disparate tooling decreases the data team’s productivity, inhibiting innovation.
Though a Cloud Data Warehouse like Snowflake is purpose-built for high and complex BI workloads, for a typical warehouse in a large enterprise, the BI workload typically comprises only 25% of the overall load, while a significant chunk of workloads includes data ingestion and engineering activities combined.
Statista forecasts 181 Zettabytes of data by 2025. With the ever-growing volume of enterprise and external data, the need for high compute, scalable platforms that can cater to modern, future-looking use cases and AI-infused business processes grows. We have seen that when enterprises start their data platform modernization journey with a cloud data warehouse, the cost differences of data processing are not significant at the lower volume. However, when the volume of data grows from the Gigabyte to the Terabyte range, the cost of data transformation becomes apparent. A premium BI engine being utilized for data processing – may not be a smart decision in the long run.
Databricks provides data handling cost advantages at 3 levels:
- Data Loading: Cloud-native storage without data staging avoids extra copies of data, saving processing time and cost.
- Processing Engine: Delta Lake and Photon are designed to enable fast and cost-efficient data processing. Whether handling SQL queries or AI and data engineering tasks, Databricks provides these powerful technologies readily available.
- ETL at Scale: Databricks has an architectural advantage with auto-scaling, Delta Live Tables (DLT), and task-level orchestration.
Why Migrate from Snowflake to Databricks?
The Databricks Lakehouse platform provides a single source of truth for all your data with end-to-end ETL and streaming, native or industry-leading high-performant BI, and first-class AI/ML on your data lake with open and unified governance and security for all your data and associated assets.
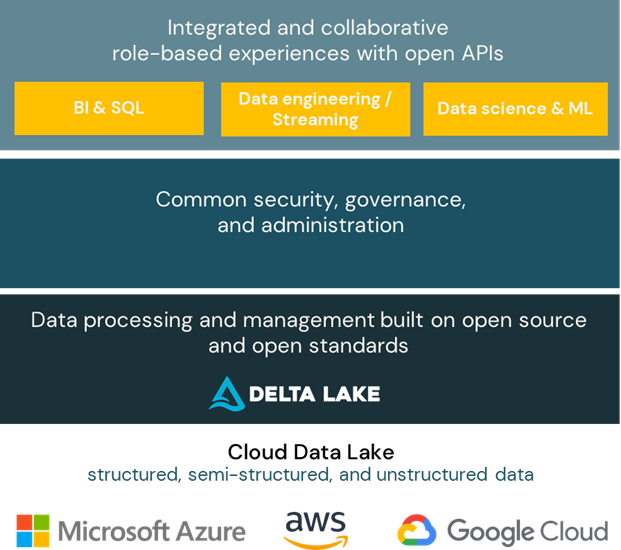
Source: Databricks
The major advantages of the Databricks Lakehouse platform are:
- Unified Data Platform: Databricks provides a unified platform for both data engineering and data science, allowing organizations to handle data at all stages – ingestion, transformation, analysis, and machine learning (ML) – without switching between different tools. This capability fosters collaboration among data engineers, data scientists, and analysts, driving faster insights and innovation. The unified platform democratizes value creation through a self-service experience bounded by an organization-wide data governance strategy with the Unity Catalog.
- Real-Time Streaming: Databricks’ open ecosystem supports multiple streaming sources with native cloud storage. However, most cloud data warehouses like Snowflake are inflexible and typically limited to Kafka streaming with high latency.
- Advanced Machine Learning and AI Capabilities: While Snowflake excels in data warehousing, Databricks offers superior machine learning and AI capabilities. Built on Apache Spark, Databricks provides an environment optimized explicitly for ML workflows, including GPU support, MLflow integration, and a vast library of ML and deep learning tools. Organizations developing sophisticated ML models may find Databricks more aligned with their goals. Databricks caters to the full suite of AI-ML offerings from data preparation to feature engineering, notebook processing for small and large datasets with multiple nodes, low code Auto-ML, batch and real-time inference. Snowflake requires third-party software integration for some of these offerings for high-performance requirements.
- Cost-Effective Data Storage and Processing: Databricks allows organizations to store and process data using open-source file formats like Delta Lake, which can be significantly more cost-effective than Snowflake’s proprietary data format. Additionally, Databricks offers the flexibility to scale up and down based on workload requirements, potentially reducing the compute cost. This platform is built to scale and optimize performance and cost. The Lakehouse architecture enables curating data that offers trusted Data-as-Products while removing data silos and minimizing data movements.
- Open-Source Ecosystem and Integration: Databricks is highly compatible with various open-source data tools, formats, and frameworks, making it easier for organizations to integrate with their existing tech stack. This level of flexibility is invaluable for enterprises invested in open-source technologies.
- Flexible Data Lakehouse Architecture: Databricks’ Lakehouse architecture merges the best features of data lakes and data warehouses, providing a single platform that supports structured and unstructured data. This architecture can simplify data governance, reduce data silos, and improve data consistency across the organization. Most importantly, the Databricks Lakehouse, a polyglot technology – can support any data model at all scales:
- Dimension Modeling: Raw/stage – Enterprise ODS/integration – Data Mart/Presentation
- Data Vault 2.0: Raw – Enterprise ODS of Hub/Link/Satellite – Business View
- Data Mesh: Data Domain (Producer/Consumer)
Strategy Levers for Snowflake to Databricks Migration
Migrating from Snowflake to Databricks involves careful planning and execution to ensure a seamless transition with minimal disruption to business operations. Two broader high-level strategies are recommended for Snowflake to Databricks migration:
- Quick TCO savings: Aiming minimal disruption to the business – leading with the migration of ETL/ELT workloads while retaining Snowflake for the serving (in the interim) of the consumption workloads and then slowly migrating the dashboards and apps to be served from the Databricks Lakehouse. This helps bring Databricks for data ingestion and engineering works, saving costs.
- Quick Value Unlock: Onboarding X-functional teams to Databricks by modernizing the report layer while keeping the GOLD data in sync with Snowflake and reconfiguring the ETL/ELT workloads in parallel. This strategy opens up the value of unlocking an organization’s data assets by democratizing data insights and collaboration.
How WinWire Can Help?
Partnering with WinWire as a Systems Integrator (SI) offers key advantages for your migration
- Expertise and Experience: WinWire helps identify inefficiencies, pain points, and unserved use cases in your current architecture. We leverage our experience in cloud-to-Databricks migrations to ensure best practices are followed.
- Customized Migration Strategy: We create tailored migration strategies based on your business needs, whether phased migration, parallel run, or big-bang cutover.
- Tools and Accelerators: Our proprietary tools, AIDQ and Migration as a Service (MaaS), accelerate the migration process, reducing costs and time.
- Risk Management and Compliance: We address risks like data loss or downtime to ensure secure, compliant migration.
- Training and Change Management: We provide training to upskill teams on Databricks and ensure a smooth user transition.
- Post-Migration Support: WinWire offers ongoing support to optimize performance and manage data workloads post-migration.
Connect with us today to discover how WinWire can seamlessly guide your Snowflake to Databricks migration, unlocking enhanced data performance and agility!