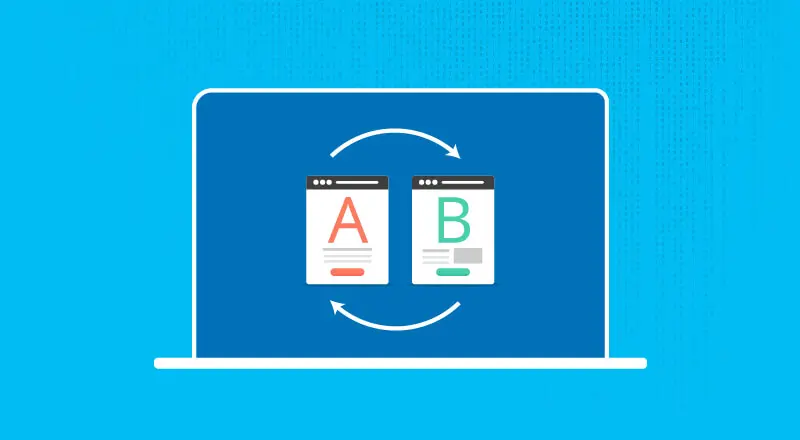
What is A/B Testing?
The A/B Testing is a statistical method that help to determines which of the two versions will produces the most excellent result based on hypothetical situation. In A/B testing, A signifies to ‘Control’ or the original testing variable. Although B refers to ‘Variation’ or a new version of the primary testing variable. The version which is closer to our business metric(s) with encouraging result, same version is concluded as the final or best solution.
A/B testing is a wonderful technique to calculate the best online promotional and marketing strategies for our business endeavours.
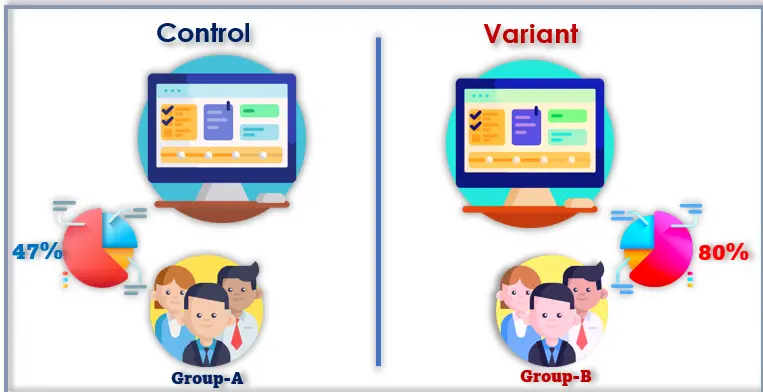
What is the Need of A/B Testing?
A/B testing help stakeholders to identify the answers:
“Do Customer preferred -Option X or Option Y”
The answer to this query is essential in the world of digital products, for variety of reasons including “Strengthening Marketing Strategies and Enhancing the Customer Experience”.
The objective of A/B testing is to optimize the current state to see the best way is to do an activity or validate an idea to see which activity is the best for better experience. Also, if there is a metric that you want to improve or you see a metric which is not working as you anticipated, A/B testing will evolve solution that you apply to fix the problem. This will also help in make improved product and apply effective decisions that will have a great influence on end user experience.
If we consider A/B testing as a solution, this will help us in-
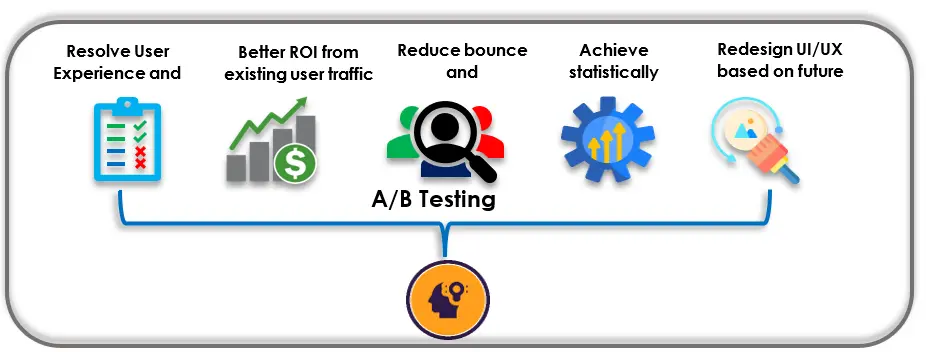
How A/B Testing optimized Product or Website?
By using data analysis and user feedback, A/B testing will assist product managers or stakeholders in obtaining information to decide how to enhance customer experiences with brand new products. We may also predict whether the new features are being used as intended and whether they are efficient by employing for A/B testing. We can get all the valuable information by monitoring user activity on website and contrasting user actions over numerous iterations and navigations. By refining the product metrics, we can optimize the user output, involvement and reliability by learning from user data statistics and behaviour.
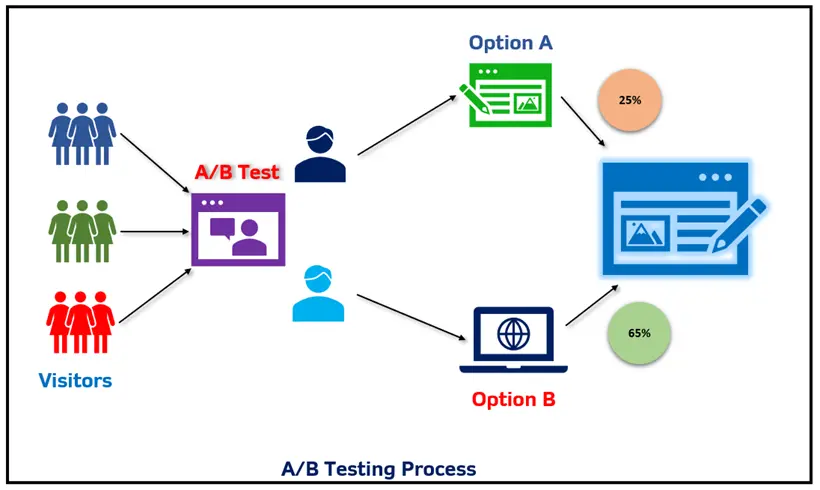
How to perform an A/B test?
A/B testing is used to determine what aspects of a marketing campaign work effectively and poorly based on certain hypothesis and data. The majority of marketing initiatives aims to boost the website user traffic. As acquiring internet traffic becomes more challenging and expensive, it is crucial to give your visitors the best experience possible. This will help them accomplish their aspirations and provide them the best possibility to convert potential customers. A/B testing in marketing makes it feasible to maximise your present traffic and increase revenue.
A/B Testing Process lifecycle-
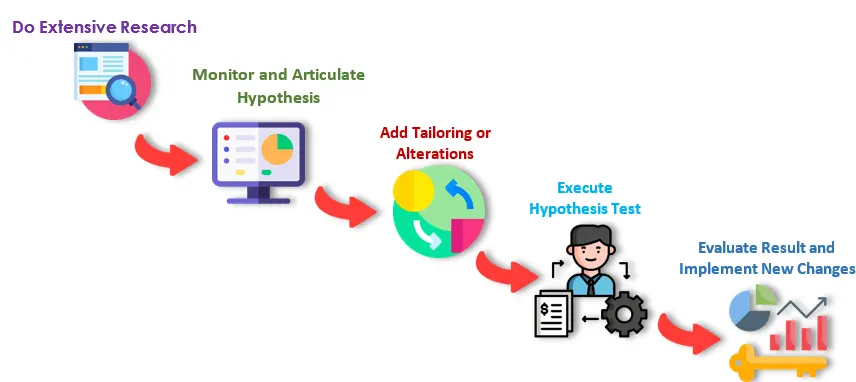
Generally, this includes the below process
Do Extensive Research
We must thoroughly investigate the website’s existing performance prior to developing an A/B testing strategy. We need to gather the information on the number of visitors to the site, the most popular pages, conversion of site. Using website analytics tools like Google Analytics, Omniture, , and others, you can identify your most popular pages, sites where users spend the most time, and pages with the lowest bounce rates. The most popular method for figuring out what visitors are scrolling to, where they are spending the most time, etc. is called a heatmap tool. This might aid in locating trouble spots on your website.
Monitor and Articulate Hypothesis
By documenting research findings and developing a conversion boosting hypotheses based on backend data, we may get closer to our company metrics objectives. Our test campaign should be aligned and scheduled as per the company goals and data collected using the qualitative and quantitative research. This will help us in gathering visitor behaviour and navigation data. The effective way to make use of data is to study and analyse accurately, make careful observations, and use websites insights to create hypotheses that are supported by the statistics. When the hypothesis is ready, test it using a variety of scenarios, including your level of confidence variable and how this will affect targeted audience at larger level while employing.
Add Tailoring or Alterations
Our testing should proceed with adding a variant into our hypothesis and apply A/B test comparing to the current version. A variation is an additional tailoring of your current iteration that includes modifications that you want to test. To determine which variant is performs most excellent, test varies from one iteration to other against the control version with continues alteration, till the right outcome is not achieved. Based on our analysis we may add functions or UI/UX designs to create a next level variant.
Execute Hypothesis Test
Decide on the sort of testing methodology and method we wish to utilise before moving on to the execution phase. Once we have decided which of these types and techniques is best fits for website requirements and commercial objectives, start executing Hypothesis test and wait till allotted or schedule time for statistically significant results. Whatever approach we use, keep in mind that the final findings will depend on our testing strategy and statistical precision that we have applied.
Calculate the complete test duration with an average Daily, Weekly and monthly visitors, projected existing conversion rate, minimum improvement in conversion rate, number of variations, proportion of visitors included in the test for effective results.
Evaluate Results and Implement new changes
Hypothesis test report showcase the outcomes which is the final stage in determining our campaign’s test result and solution with improvement metrics. This is the stage where we can analyse the entire test unravels with A/B testing necessitates ongoing data collection and analysis. Analyse the findings when the test is finished by considering indicators like percentage increase, confidence level in data, direct and indirect influence on other metrics. After weighing these figures, if the test is successful, implement the new changes for the winning variant. Draw the conclusion from the report and use for stakeholders to showcase the test result and implementation.
Different Types of A/B Testing
There are many types of testing methodologies and their benefits after learning about which web page sections to test to improve our product and website metrics. Besides conventional A/B testing, there are three kinds of A/B tests that can be leveraged depending on the situation:
A/B testing offers three types of testing fundamentally to complete the Hypothesis-
- Split URL Testing
- Multivariate Testing
- Multipage Testing
Common Mistakes of A/B Testing
A/B testing can yield insightful information and inaccurate outcomes can distort results and force teams to base critical choices on suspect information.
A/B testing errors that are more typical include and that we can avoid-
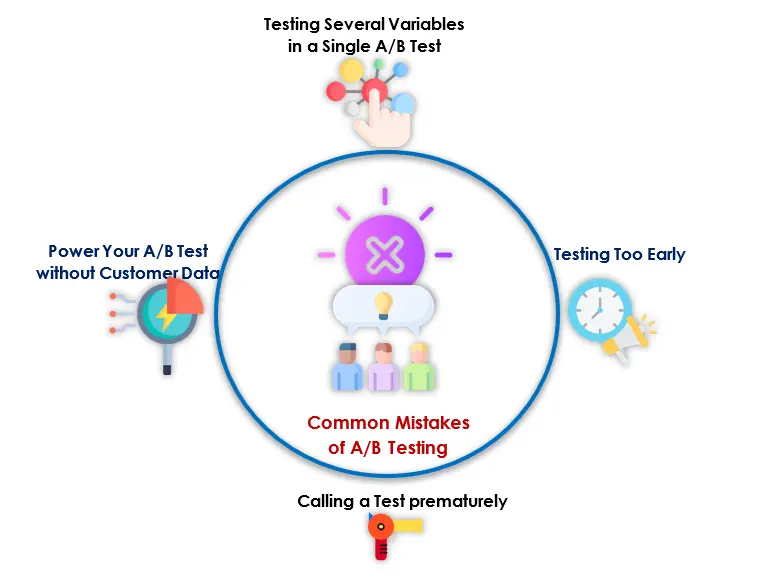
- Testing Several Variables in a Single A/B Test
One variable at a time is tested in an A/B test. By limiting the A and B choices to a single change allows us to know with certainty that this specific change was responsible for the result.
- Testing Too Early
We can’t evaluate changes against the appropriate data without a baseline that has been set. It pays to put off A/B testing for a long if we simply built up a new landing page.
- Calling a Test Prematurely
Based on encouraging outcomes, we could be tempted to declare victory after just a few days. A more accurate picture of how consumers will respond to your modifications over time may be obtained by conducting testing over a period of several weeks.
- Power Your A/B Tests without Customer Data
In large-scale A/B testing, historical, demographical, and behavioural data are employed. Without data, the results will offer you erroneous numbers and wrong observations.
Conclusion
By assessing user behaviour in real-time, A/B testing responds to and meets users’ demands. We may implement all the features that emphasise the greatest user experience after analysing the findings of A/B testing. As QA testers, we have to create a checklist and check the various product iterations and data gathering techniques for flaws to ensure that the tests run well. A/B testing may be used by the QA team and developers to build products that are user-friendly and matches the company metrics and goals.
Making better product decisions is aided by testing experiments, therefore A/B testing may be thought of as an advice backed by the evidence.